Biomineralization
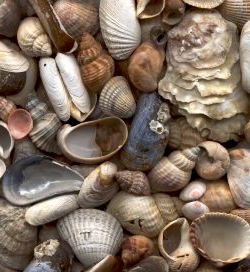
Biominerals are known to exhibit outstanding properties. Different morphologies and different shapes arranged in superstructures allow for a great variety of properties. How and when these structural features are encoded along the crystallization pathway is still largely unknown. While analyzing the macroscopic result of crystallization is quite easy, gaining insight into the early stages of crystallization through experimentation is difficult. The small size of these early stage structures, which is challenging for many experimental techniques on the one hand, is an advantage for molecular dynamics (MD) simulations on the other hand.
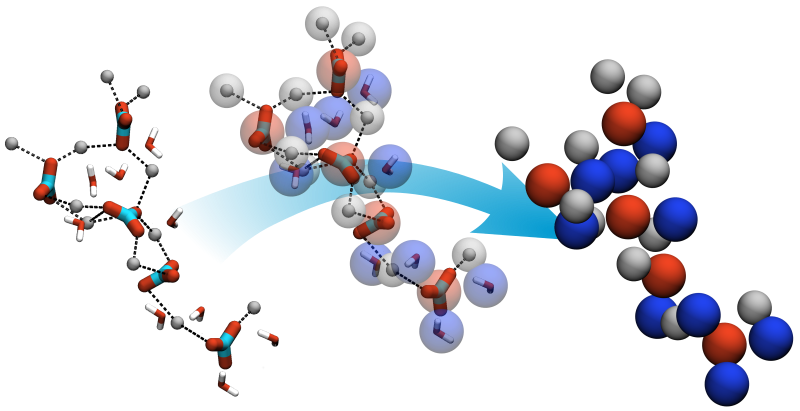
Calcium minerals can be found in various materials, like teeth, bone and shells, but also be used or occur in industrial processes. The formation of these materials can be addressed by MD simulation on different resolution levels but is computational nevertheless expensive. For insights on the early stages of nanoparticle formation and growth we develop particle-based coarse-grained models for Calcium minerals.

Some of the main components of biominerals besides the mineral itself are macromolecules, which appear to be the key for understanding their structural variety. Macromolecules isolated from calcium carbonate biominerals typically contain parts rich in aspartic acid. The influence of this amino acid on mineral formation can be explained with the ability to bind to metal ions with the negatively charged carboxylate group in the side chain.
Simulations of such mineralization modifiers suffer from slow sampling due to high energetic barriers caused by strong ion bridges. Even with the application of enhanced sampling techniques like Hamiltonian replica exchange and Metadynamics sampling to convergence remains a challenge. To tackle these sampling problems, the application of machine learning techniques is evaluated.

Biomineralization is the intricate process employed by living organisms to form minerals. Human orthopedic conditions, such as osteoporosis, are a direct consequence of poorly orchestrated biomineralization. Deciphering the molecular mechanism of this vital yet poorly understood process is thus essential for the development of therapeutic approaches. Using multiples simulations on amphiphilic peptides we identified the effects of various aspects of the peptide sequence (length, terminal, side-chains) on aggregate stability and ion-peptide interactions.